Axnn Energy Efficient Neuromorphic Systems Using Approximate Computing
We propose a new approach to design energy-efficient hardware implementations of large-scale neural networks NNs using approximate computing. Energy-efficient neuromorphic systems using approximate computing.
Axnn Energy Efficient Neuromorphic Systems Using Approximate Computing Semantic Scholar
International Symposium on Low Power Electronics and Design pp.
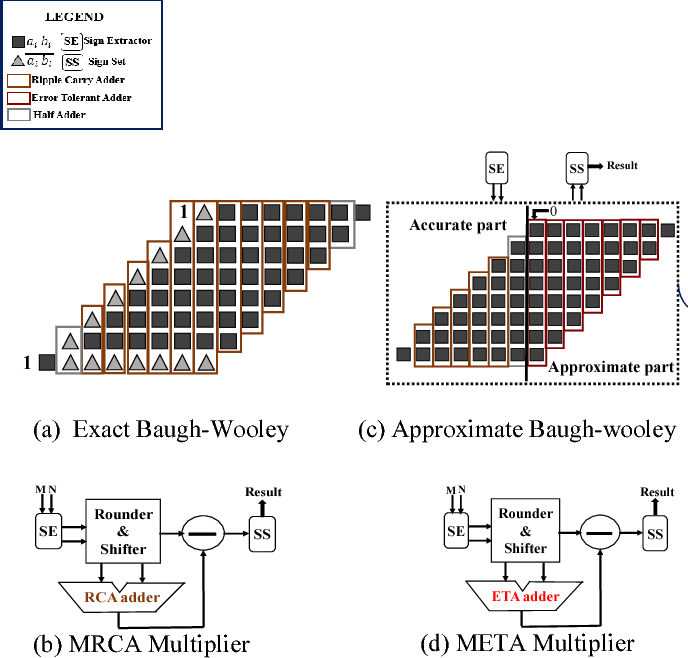
Axnn energy efficient neuromorphic systems using approximate computing. Approximate computing leverages the intrinsic error tolerance of a neural network for improved energy efficiencyThe main objective is maintaining good enough accuracy with aggressive approximation. Energy-efficient neuromorphic systems using approximate computing Abstract. 1.
Watson Research Center - Dikutip 831 kali - AI Hardware Architectures - Approximate Computing - Spintronic Memory Architectures Memuat. Learning in Energy-Efficient Neuromorphic Computing. Research Staff Member IBM TJ.
Our work is motivated by the observations that i NNs are used in applications where less-than-perfect results are acceptable and often inevitable and ii they are highly resilient to inexactness in many but not all of their constituent computations. XUIMIQQOPSSXEZ-UHFFFAOYSA-N silicon Chemical compound XUIMIQQOPSSXEZ-UHFFFAOYSA-N. Algorithm and Architecture Co-Design is an ideal resource for researchers scientists software engineers and hardware engineers dealing with the ever-increasing requirement on power consumption and response time.
Energy- efficient neuromorphic systems using approximate computing. Purdue University West lafayette IN USA. AxNN provides a method for improving energy efficiency of neural networks by approximating neurons that do not contribute to output error.
Anand Raghunathan Northwestern University Purdue University A Model for Arraybased Approximate Arithmetic Computing with Application to. 210000000653 nervous system Anatomy 0000 description 3. Our work is motivated by the observations that i NNs are used in applications where less-than-perfect results are acceptable and often inevitable and ii they are highly resilient to inexactness in many but not all of their constituent.
An Approximate Computing Framework for Artificial Neural Network Qian Zhang Ting Wang Ye Tian Feng Yuan and Qiang Xu CUhk REliable computing laboratory CURE Department of Computer Science Engineering The Chinese University of Hong Kong Shatin NT Hong Kong Email. 230000000306 recurrent Effects 0000 description 3. 210000002569 neurons Anatomy 0000 description 3.
230000002104 routine Effects 0000 description 3. We propose a new approach to design energy-efficient hardware implementations of large-scale neural networks NNs using approximate computing. Energy-efficient neuromorphic systems using approximate computing.
Venkataramani S Ranjan A Roy K Raghunathan A. Energy-efficient neuromorphic systems using approximate computing articleVenkataramani2014AxNNEN titleAxNN. Energy-efficient neuromorphic systems using approximate computing.
Neuromorphic algorithms which are comprised of highly complex large-scale networks of artificial neurons are increasingly used for a variety of recognition classification search and vision tasks. Energy Efficient Neuromorphic Systems using Approximate Computing Yigit Demir Nikos Hardavellas Swagath Venkataramani. Energy-efficient neuromorphic systems using approximate computing authorSwagath Venkataramani and Ashish Ranjan and K.
QC-NPE hardware platform to efficiently execute AxNNs. Neuromorphic algorithms which are comprised of highly complex large-scale networks of artificial. Home Conferences ISLPED Proceedings ISLPED 14 AxNN.
Energy-efficient neuromorphic systems using approximate computing. Energy-efficient neuromorphic systems using approximate computing. It is also excellent for teaching and training undergraduate and graduate students about the latest generation.
Raghunathan journal2014 IEEEACM International Symposium on Low. In this paper we propose the AxTrain framework to. Energy-Efficient Neuromorphic Systems using Approximate Computing Swagath Venkataramani Ashish Ranjan Kaushik Roy and Anand Raghunathan School of Electrical and Computer Engineering Purdue University venkata0aranjankaushikraghunathanpurdueedu ABSTRACT Neuromorphic algorithms which are comprised of highly complex large-scale networks of artificial neurons are increasingly used for a variety of recognition classification search and.
2732 2014 Google Scholar. Energy-efficient neuromorphic systems using approximate computing.
Axnn Energy Efficient Neuromorphic Systems Using Approximate Computing Semantic Scholar
Data And Power Efficient Intelligence With Neuromorphic Learning Machines Iscience
Figure 2 From Axnn Energy Efficient Neuromorphic Systems Using Approximate Computing Semantic Scholar
Approximate Computing Methods For Embedded Machine Learning Semantic Scholar
Axnn Energy Efficient Neuromorphic Systems Using Approximate Computing Semantic Scholar
Figure 1 From Axnn Energy Efficient Neuromorphic Systems Using Approximate Computing Semantic Scholar
Pdf Energy Efficient Convnets Through Approximate Computing Semantic Scholar
Pdf Energy Efficient Convnets Through Approximate Computing Semantic Scholar
Axnn Energy Efficient Neuromorphic Systems Using Approximate Computing Semantic Scholar
Axnn Energy Efficient Neuromorphic Systems Using Approximate Computing Semantic Scholar
Approximate Computing A Survey Of Recent Trends Bringing Greenness To Computing And Communication Springerlink
Https Ascslab Org Papers Approximate Pdf
Pdf Approximate Computing A Survey Of Recent Trends Bringing Greenness To Computing And Communication
Approximate Computing Methods For Embedded Machine Learning Semantic Scholar
Https Ieeexplore Ieee Org Iel7 9179985 9180369 09180840 Pdf
Pdf Energy Efficient Convnets Through Approximate Computing Semantic Scholar
Electronics Free Full Text Accelerating Neural Network Inference On Fpga Based Platforms A Survey Html
Pdf Energy Efficient Convnets Through Approximate Computing Semantic Scholar
Pdf Energy Efficient Convnets Through Approximate Computing Semantic Scholar
Post a Comment for "Axnn Energy Efficient Neuromorphic Systems Using Approximate Computing"